Data Mining by Mehmed Kantardzic (inspirational novels TXT) 📗
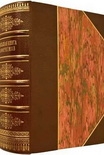
- Author: Mehmed Kantardzic
Book online «Data Mining by Mehmed Kantardzic (inspirational novels TXT) 📗». Author Mehmed Kantardzic
It is not only that DDM infrastructure is changing by offering new approaches through Web services together with the grid technology. Basic data-mining algorithms also need changes in a distributed environment. Most off-the-shelf data-mining systems are designed to work as a monolithic centralized application. They normally download the relevant data to a centralized location and then perform the data-mining operations. This centralized approach does not work well in many of the emerging distributed, ubiquitous, possibly privacy-sensitive data-mining applications. A primary goal of DDM algorithms is to achieve the same or similar data-mining result as a centralized solution without moving data from their original locations. The distributed approach assumes that local computation is done on each of the sites, and either a central site communicates with each distributed site to compute the global model, or a peer-to-peer architecture is used. In the latter case, individual nodes perform most of the tasks by communicating with neighboring nodes by message passing over an asynchronous network. Illustrative examples are networks of independent and intelligent sensors that are connected to each other in an ad hoc fashion. Some features of a distributed mining scenario are as follows:
The system consists of multiple independent sites of data and computation.
Sites exchange their results by communicating with other sites, often through message passing.
Communication between the sites is expensive and often represents a bottleneck.
Sites have resource constraints, for example, battery power in distributed sensors systems.
Sites have privacy and/or security concerns.
The system should have the ability to efficiently scale up because distributed systems today may consist of millions of nodes.
The system should have the ability to function correctly in the presence of local site failures, and also missing or incorrect data.
Obviously, the emphasis in DDM algorithms is on local computation and communication. Local algorithms for DDM can be broadly classified under two categories:
Exact Local Algorithms. These algorithms guarantee to always terminate with precisely the same result that would have to be found by a centralized algorithm. Exact local algorithms are obviously more desirable but are more difficult to develop, and in some cases seemingly not possible.
Approximate Local Algorithms. These algorithms cannot guarantee accuracy by centralized solutions. They make a balance between quality of solution and system’s responses.
Selection of a type of a local algorithm depends on the data-mining problem and application domain, including the amount of data and their dynamics. In general, approximate approaches are used in cases when the balance between accuracy and efficiency is important, and communications between sites represent a bottleneck. We will illustrate this balance between local computation and communication with a simple approximate algorithm useful in many data-mining applications. For example, if we want to compare the data vectors observed at different sites, the centralized approach will collect these vectors to the central computer and then compare the vectors using whatever metric is appropriate for the domain. DDM technology offers more efficient solutions for the problem using a simple randomized technique.
Vectors a = (a1, a2, … , am) and b = (b1, b2, … , bm) are given at two distributed sites A and B, respectively. We want to approximate the Euclidean distance between them using a small number of messages and reduced data transfer between sites A and B. Centralized solution requires that one vector is transferred to the other site, that is, m components of one vector are transferred. How does one obtain the same result with less than m data transfer? Note that the problem of computing the Euclidean distance between a pair of vectors a and b can be represented as the problem of computing the inner products as follows:
where (a • b) represents a inner product between vectors a and b defined
Comments (0)