Data Mining by Mehmed Kantardzic (inspirational novels TXT) 📗
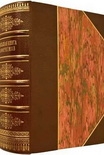
- Author: Mehmed Kantardzic
Book online «Data Mining by Mehmed Kantardzic (inspirational novels TXT) 📗». Author Mehmed Kantardzic
Usually X is referred to as the universe of discourse, or, simply, the universe, and it may consist of discrete (ordered or non-ordered) objects or continuous space. This can be clarified by the following examples. Let X = {San Francisco, Boston, Los Angeles} be the set of cities one may choose to live in. The fuzzy set C = “desirable city to live in” may be described as follows:
The universe of discourse X is discrete and it contains non-ordered objects: three big cities in the United States. As one can see, the membership grades listed above are quite subjective; anyone can come up with three different but legitimate values to reflect his or her preference.
In the next example, let X = {0, 1, 2, 3, 4, 5, 6} be a set of the number of children a family may choose to have. The fuzzy set A = “sensible number of children in a family” may be described as follows:
Or, in the notation that we will use through this chapter,
Here we have a discrete-order universe X; the MF for the fuzzy set A is shown in Figure 14.1a. Again, the membership grades of this fuzzy set are obviously subjective measures.
Figure 14.1. Discrete and continuous representation of membership functions for given fuzzy sets. (a) A = “sensible number of children”; (b) B = about 50 years old.
Finally, let X = R+ be the set of possible ages for human beings. Then the fuzzy set B = “about 50 years old” may be expressed as
where
This is illustrated in Figure 14.1b.
As mentioned earlier, a fuzzy set is completely characterized by its MF. Since many fuzzy sets in use have a universe of discourse X consisting of the real line R, it would be impractical to list all the pairs defining an MF. A more convenient and concise way to define an MF is to express it as a mathematical formula. Several classes of parametrized MFs are introduced, and in real-world applications of fuzzy sets the shape of MFs is usually restricted to a certain class of functions that can be specified with only few parameters. The most well known are triangular, trapezoidal, and Gaussian; Figure 14.2 shows these commonly used shapes for MFs.
Figure 14.2. Most commonly used shapes for membership functions. (a) Triangular; (b) trapezoidal; (c) Gaussian.
A triangular MF is specified by three parameters {a, b, c} as follows:
The parameters {a, b, c}, with a < b < c, determine the x coordinates of the three corners of the underlying triangular MF.
A trapezoidal MF is specified by four parameters {a, b, c, d} as follows:
The parameters {a, b, c, d}, with a < b ≤ c < d, determine the x coordinates of the four corners of the underlying trapezoidal MF. A triangular MF can be seen as a special case of the trapezoidal form where b = c.
Finally, a Gaussian MF is specified by two parameters {c, σ}:
A Gaussian MF is determined completely by c and σ; c represents the membership-function center, and σ determines the membership-function width. Figure 14.3 illustrates the three classes of parametrized MFs.
Figure 14.3. Examples of parametrized membership functions. (a) Triangle(x, 20, 60, 80); (b) trapezoid(x, 10, 20, 60, 90); (c) Gaussian(x, 50, 20).
From the preceding examples, it is obvious that the construction of a fuzzy set depends on two things: the identification of a suitable universe of discourse and the specification of an appropriate MF. The specification of an MF is subjective, which means that the MFs for the same concept (e.g., “sensible number of children in a family”) when specified by different persons may vary considerably. This subjectivity comes from individual differences in perceiving or expressing abstract concepts and has little to do with randomness. Therefore, the subjectivity and nonrandomness of fuzzy sets is the primary difference between the study of fuzzy sets and the probability theory, which deals with the objective treatment of random phenomena.
There are several parameters and characteristics of MF that are used very often in some fuzzy-set operations and fuzzy-set inference systems. We will define only some of them that are, in our opinion, the most important:
1. Support. The support of a fuzzy set A is the set of all points x in the universe of discourse X such that μA(x) > 0:
2. Core. The core of a fuzzy set A is the set of all points x in X such that μA(x) = 1:
3. Normalization. A fuzzy set A is normal if its core is nonempty. In other words, we can always find a point x ∈ X such that μA(x) = 1.
4. Cardinality. Given a fuzzy set A in a finite universe X, its cardinality, denoted by Card(A), is defined as
Often, Card(X) is referred to as the scalar cardinality or the count of A. For example, the fuzzy set A = 0.1/1 + 0.3/2 + 0.6/3 + 1.0/4 + 0.4/5 in universe X = {1, 2, 3, 4, 5, 6} has a cardinality Card(A) = 2.4.
5. α-cut. The α-cut or α-level set of a fuzzy set A is a crisp set defined by
6. Fuzzy number. Fuzzy numbers are a special type of fuzzy sets restricting the possible types of MFs:
(a) The MF must be normalized (i.e., the core is nonempty) and singular. This results in precisely one point, which lies inside the core, modeling the typical value of the fuzzy number.
Comments (0)