Data Mining by Mehmed Kantardzic (inspirational novels TXT) 📗
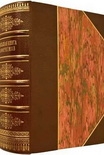
- Author: Mehmed Kantardzic
Book online «Data Mining by Mehmed Kantardzic (inspirational novels TXT) 📗». Author Mehmed Kantardzic
There are various strategies for drawing a representative subset of samples from a data set. The size of a suitable subset is determined by taking into account the cost of computation, memory requirements, accuracy of the estimator, and other characteristics of the algorithm and data. Generally, a subset size can be determined so that the estimates for the entire data set do not differ by more than a stated margin error in more than δ of the samples. By setting up a probability inequality P(|e − e0| ≥ ε) ≤ δ, we solve it for the subset of sample size n, and for a given value ε (confidence limit) and δ (where 1 − δ is the confidence level). The parameter e stands for an estimate from the subset and it is generally a function of the subset size n, while e0 stands for the true value obtained from entire data set. However, e0 is usually unknown too. In this case, a practical way to determine the required size of the data subset can be done as follows: In the first step we select a small preliminary subset of samples of size m. Observations made based on this subset of data will be used to estimate e0. After replacing e0 in the inequality, it is solved for n. If n ≥ m, additional n − m samples are selected in the final subset for analysis. If n ≤ m no more samples are selected, and the preliminary subset of data is used as the final.
One possible classification of sampling methods in data mining is based on the scope of application of these methods, and the main classes are
1. general-purpose sampling methods
2. sampling methods for specific domains.
In this text we will introduce only some of the techniques that belong to the first class because they do not require specific knowledge about the application domain and may be used for a variety of data-mining applications.
Systematic sampling is the simplest sampling technique. For example, if we want to select 50% of a data set, we could take every other sample in a database. This approach is adequate for many applications and it is a part of many data-mining tools. However, it can also lead to unpredicted problems when there are some regularities in the database. Therefore, the data miner has to be very careful in applying this sampling technique.
Random sampling is a method by which every sample from an initial data set has the same chance of being selected in the subset. The method has two variants: random sampling without replacement and random sampling with replacement. Random sampling without replacement is a popular technique in which n distinct samples are selected from N initial samples in the data set without repetition (a sample may not occur twice). The advantages of the approach are simplicity of the algorithm and nonexistence of any bias in a selection. In random sampling with replacement, the samples are selected from a data set such that all samples are given an equal chance of being selected, no matter how often they already have been drawn, that is, any of the samples may be selected more than once. Random sampling is not a one-time activity in a data-mining process. It is an iterative process, resulting in several randomly selected subsets of samples. The two basic forms of a random sampling process are as follows.
1. Incremental Sampling. Mining incrementally larger random subsets of samples that have many real-world applications helps spot trends in error and complexity. Experience has shown that the performance of the solution may level off rapidly after some percentage of the available samples has been examined. A principal approach to case reduction is to perform a data-mining process on increasingly larger random subsets, to observe the trends in performances, and to stop when no progress is made. The subsets should take big increments in data sets, so that the expectation of improving performance with more data is reasonable. A typical pattern of incremental subsets might be 10, 20, 33, 50, 67, and 100%. These percentages are reasonable, but can be adjusted based on knowledge of the application and the number of samples in the data set. The smallest subset should be substantial, typically, no fewer than 1000 samples.
2. Average Sampling. When the solutions found from many random subsets of samples of cases are averaged or voted, the combined solution can do as well or even better than the single solution found on the full collection of data. The price of this approach is the repetitive process of data mining on smaller sets of samples and, additionally, a heuristic definition of criteria to compare the several solutions of different subsets of data. Typically, the process of voting between solutions is applied for classification problems (if three solutions are class1 and one solution is class2, then the final voted solution is class1) and averaging for regression problems (if one solution is 6, the second is 6.5, and the third 6.7, then the final averaged solution is 6.4). When the new sample is to be presented and analyzed by this methodology, an answer should be given by each solution, and a final result will be obtained by comparing and integrating these solutions with the proposed heuristics.
Two additional techniques, stratified sampling and inverse sampling, may be convenient for some data-mining applications. Stratified sampling is a technique in which the entire data set is split into nonoverlapping subsets or strata, and sampling is performed for each different stratum independently of another. The combination of all the small subsets from different strata forms the final, total subset of data samples for analysis. This technique is used when the strata are relatively homogeneous and the variance of the overall estimate is smaller than that
Comments (0)