Data Mining by Mehmed Kantardzic (inspirational novels TXT) 📗
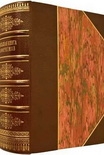
- Author: Mehmed Kantardzic
Book online «Data Mining by Mehmed Kantardzic (inspirational novels TXT) 📗». Author Mehmed Kantardzic
Free e-book «Data Mining by Mehmed Kantardzic (inspirational novels TXT) 📗» - read online now
Similar e-books:
Comments (0)