Data Mining by Mehmed Kantardzic (inspirational novels TXT) 📗
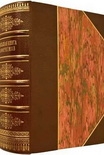
- Author: Mehmed Kantardzic
Book online «Data Mining by Mehmed Kantardzic (inspirational novels TXT) 📗». Author Mehmed Kantardzic
B.2 DATA MINING FOR THE TELECOMUNICATIONS INDUSTRY
The telecommunication industry has quickly evolved from offering local and long-distance telephone services to providing many other comprehensive communication services including voice, fax, pager, cellular phone, images, e-mail, computer, and Web-data transmission, and other data traffic. The integration of telecommunications, computer networks, Internet, and numerous others means of communication and computing is under way. The U.S. Telecommunication Act of 1996 allowed Regional Bell Operating Companies to enter the long-distance market as well as offer “cable-like” services. The European Liberalization of Telecommunications Services has been effective from the beginning of 1998. Besides deregulation, there has been a sale by the FCC of airwaves to companies pioneering new ways to communicate. The cellular industry is rapidly taking on a life of its own. With all this deregulation of the telecommunication industry, the market is expanding rapidly and becoming highly competitive.
The hypercompetitive nature of the industry has created a need to understand customers, to keep them, and to model effective ways to market new products. This creates a great demand for data mining to help understand the new business involved, identify telecommunication patterns, catch fraudulent activities, make better use of resources, and improve the quality of services. In general, the telecommunications industry is interested in answering some strategic questions through data-mining applications such as:
How does one retain customers and keep them loyal as competitors offer special offers and reduced rates?
Which customers are most likely to churn?
What characteristics indicate high-risk investments, such as investing in new fiber-optic lines?
How does one predict whether customers will buy additional products like cellular services, call waiting, or basic services?
What characteristics differentiate our products from those of our competitors?
Companies like AT&T, AirTouch Communications, and AMS Mobile Communication Industry Group have announced the use of data mining to improve their marketing activities. There are several companies including Lightbridge and Verizon that use data-mining technology to look at cellular fraud for the telecommunications industry. Another trend has been to use advanced visualization techniques to model and analyze wireless-telecommunication networks. Selected examples of data-mining applications in the telecommunication industry follow.
Cablevision Systems, Inc.
Cablevision Systems Inc., a cable TV provider from New York, was concerned about its competitiveness after deregulation allowed telecom companies into the cable industry. As a consequence, it decided that it needed a central data repository so that its marketing people could have faster and more accurate access to data. Using data mining, the marketing people at Cablevision were able to identify nine primary customer segments among the company’s 2.8 million customers. This included customers in the segment that are likely to “switch” to another provider. Cablevision also focused on those segments most likely to buy its offerings for new services. The company has used data mining to compare the profiles of two sets of targeted customers—those who bought new services and those who did not. This has led the company to make some changes in its messages to customers, which, in turn, has led to a 30% increase in targeted customers signing up for new services
Worldcom
Worldcom is another company that has found great value in data mining. By mining databases of its customer-service and telemarketing data, Worldcom has discovered new ways to sell voice and data services. For example, it has found that people who buy two or more services were likely to be relatively loyal customers. It also found that people were willing to buy packages of products such as long-distance, cellular-phone, Internet, and other services. Consequently, Worldcom started to offer more such packages.
BBC TV
TV-program schedulers would like to know the likely audience for a proposed program and the best time to show it. The data for audience prediction are fairly complex. Factors, which determine the audience share gained by a particular program, include not only the characteristics of the program itself and the time at which is shown, but also the nature of the competing programs in other channels. Using Clementine, Integral Solutions Limited developed a system to predict television audiences for the BBC. The prediction accuracy was reported to be the same as that achieved by the best performance of BBC’s planners.
Bell Atlantic
Bell Atlantic developed telephone technician dispatch system. When a customer reports a telephone problem to Bell Atlantic, the company must decide what type of technician to dispatch to resolve the issue. Starting in 1991, this decision was made using a hand-crafted expert system, but in 1999 it was replaced by another set of rules created with machine learning. The learned rules save Bell Atlantic more than 10 million dollars per year because they make fewer erroneous decisions. In addition, the original expert system had reached a stage in its evolution where it could not be maintained cost-effectively. Because the learned system was built by training it on examples, it is easy to maintain and to adapt to regional differences and changing cost structures.
B.3 DATA MINING FOR THE RETAIL INDUSTRY
Slim margins have pushed retailers into data warehousing earlier than other industries. Retailers have seen improved decision-support processes leading directly to improved efficiency in inventory management and financial forecasting. The early adoption of data warehousing by retailers has allowed them a better opportunity to take advantage of data mining. The retail industry is a major application area for data mining since it collects huge amounts of data on sales, customer-shopping history, goods transportation, consumption patterns, and service records, and so on.
Comments (0)